Todd S Rosenstock¹, Bjoern Ole Sander2, Klaus Butterbach-Bahl3, 4, Mariana C Rufino5, Jonathan Hickman6, Clare Stirling7, Meryl Richards8, 9, Lini Wollenberg8, 9
Abstract: This chapter explains the rationale for greenhouse gas emission estimation in tropical developing countries and why guidelines for smallholder farming systems are needed. It briefly highlights the innovations of the SAMPLES approach and explains how these advances fill a critical gap in the available quantification guidelines. The chapter concludes by describing how to use the guidelines.
Keywords: measurement guidelines, greenhouse gas emissions, carbon dioxide, nitrous oxide, methane, integrated assessment, targeting, scaling, biomass carbon, soil carbon, trace gases
Table of Contents:
1.1 Motivation for these guidelines
1.2 Who should use these guidelines
1.3 How to use these guidelines
Author affiliations
References
1.1 Motivation for these guidelines
Agriculture in tropical developing countries produces about 7-9% of annual anthropogenic greenhouse gas (GHG) emissions (Figure 1.1) and contributes to additional emissions through land use change (Smith et al. 2014). At the same time, nearly 70% of the technical mitigation potential in the agricultural sector occurs in these countries (Smith et al. 2008). Enabling farmers in tropical developing countries to manage agriculture to reduce greenhouse gas emissions intensity (emissions per unit product) is consequently an important option for mitigating future atmospheric GHG concentrations.
Our current ability to quantify GHG emissions and mitigation from agriculture in tropical developing countries is remarkably limited (Rosenstock et al. 2013). Empirical measurement is expensive and therefore limited to small areas. Emissions can be estimated for large areas with a combination of field measurement, modeling and remote sensing, but even simple data about the extent of activities is often not available and models require calibration and validation (Olander et al, 2014). These guidelines focus on how to produce field measurements as a method for consistent, robust empirical data and to produce better models.
For all but a few crops and systems, there are no measured data for the emissions of current practices or the practices that would potentially reduce net emissions. For crops, significant information has been gathered for irrigated rice systems e.g. in the Philippines, Thailand and China (Linquist et al. 2012; Siopongo et al. 2014) and for nitrous oxide emissions from China where high levels of fertilizer are applied (Ding et al. 2007; Vitousek et al. 2009). Yet measurements of methane from livestock—a major source of agricultural GHG emissions in most of the developing world—are lacking (Dickhöfer et al. 2014). Similarly, little to no information exists for most other GHG sources and sinks. Smallholder farms comprise a significant proportion of agriculture in the developing world in aggregate, as high as 98% of the agricultural land area in China, for example, yet tend to escape attention as a source of significant emissions because of the small size of individual farms.
The dearth of empirical data contributes to why most tropical developing countries, which roughly correspond to non Annex 1 countries of the UNFCCC, report emissions to the UNFCCC using Tier 1 emission factors, rather than more precise Tier 2 or Tier 3 emission factors (Ogle et al. 2014). However, Tier 1 emission factors represent a global average of data derived primarily from research conducted in temperate climates for monocultures, which is very different from the complex agricultural systems and landscapes typical of smallholder farms in the tropics. Given our knowledge of the mechanisms driving emissions and sequestration (e.g., temperature, precipitation, primary productivity, soil types, microbial activity, substrate availability), there is reason to believe that these factors represent only a rough approximation of the true values for emissions (Milne et al. 2013).
Field measurement of GHG emissions in tropical developing countries is generally done using methods developed in temperate developed countries. However, multiple factors complicate measurement of agricultural GHG sources and sinks in non-Annex 1 countries and necessitate approaches specific to the conditions common in these countries, including heterogeneity of the landscape, the need for low-cost methods, and the need for improving farmers’ livelihood and food security.
Heterogeneous landscapes Annex-1 countries are dominated by industrial agriculture, usually monocultures with commonly defined practices, over relatively large expanses. The combination of high research intensity and large-scale agriculture in developed countries creates a homogenous, relatively data-rich environment where point measurements of key sources (e.g. soil emissions from corn production in the Midwestern US or methane production from Danish dairy animals) can be extrapolated with acceptable levels of uncertainty to larger areas using empirical and process-based models (Del Grosso et al. 2008; Millar et al. 2010).
In contrast, many farmers (particularly smallholders) in tropical developing countries operate diversified farms with multiple crops and livestock, with field sizes often less than 2 ha. For example, in Western Kenya maize is often intercropped with beans, trees, or both and in regions with two rainy seasons, maize might be followed in the rotation by sorghum or other crops. Exceptions exist of course, such as in Brazil, where industrial farming is well established and farms can be thousands of hectares. Where heterogeneity does exist, it complicates the design of the sampling approach in terms of identifying the boundary of the measurement effort, stratifying the farm or landscape, and determining the necessary sampling effort. Capturing the heterogeneity of such systems, as well as comparing the effects of mitigation practices or agronomic interventions to improve productivity, often demands an impractical number of samples (Milne et al. 2013). Methods are needed to stratify complex landscapes and target measurements to the most important land units in terms of emissions and/or mitigation potential.
Resource limitations People and institutions undertaking GHG measurements have different objectives, tolerances for uncertainty, and resources. Cost of research is one of the major barriers faced by non-Annex 1 countries in moving to Tier 2 or Tier 3 quantification methods. Some methods require sampling equipment, laboratory analytical capacity, and expertise that is not available in many developing countries. Furthermore, different spatial scales (e.g. field, farm, or landscape) require different methods and approaches. The chapters in this volume guide the user in choosing from available methods, taking into account the user’s objectives, resources and capacity.
Improving livelihood and food security as a primary concern The importance of improving farmer’s livelihoods and capacity to contribute to food security through improved productivity must be taken into account in mitigation decision-making and the GHG emissions research agenda supporting those decisions. Measuring GHG emissions per unit area is a standard practice for accounting purposes, but measuring emissions per unit yield allows tracking of the efficiency of GHG for the yield produced and informs agronomic practices (Linquist et al 2012). This volume considers productivity in targeting measurements and sampling design, along with recommendations for cost-effective yield measurements.
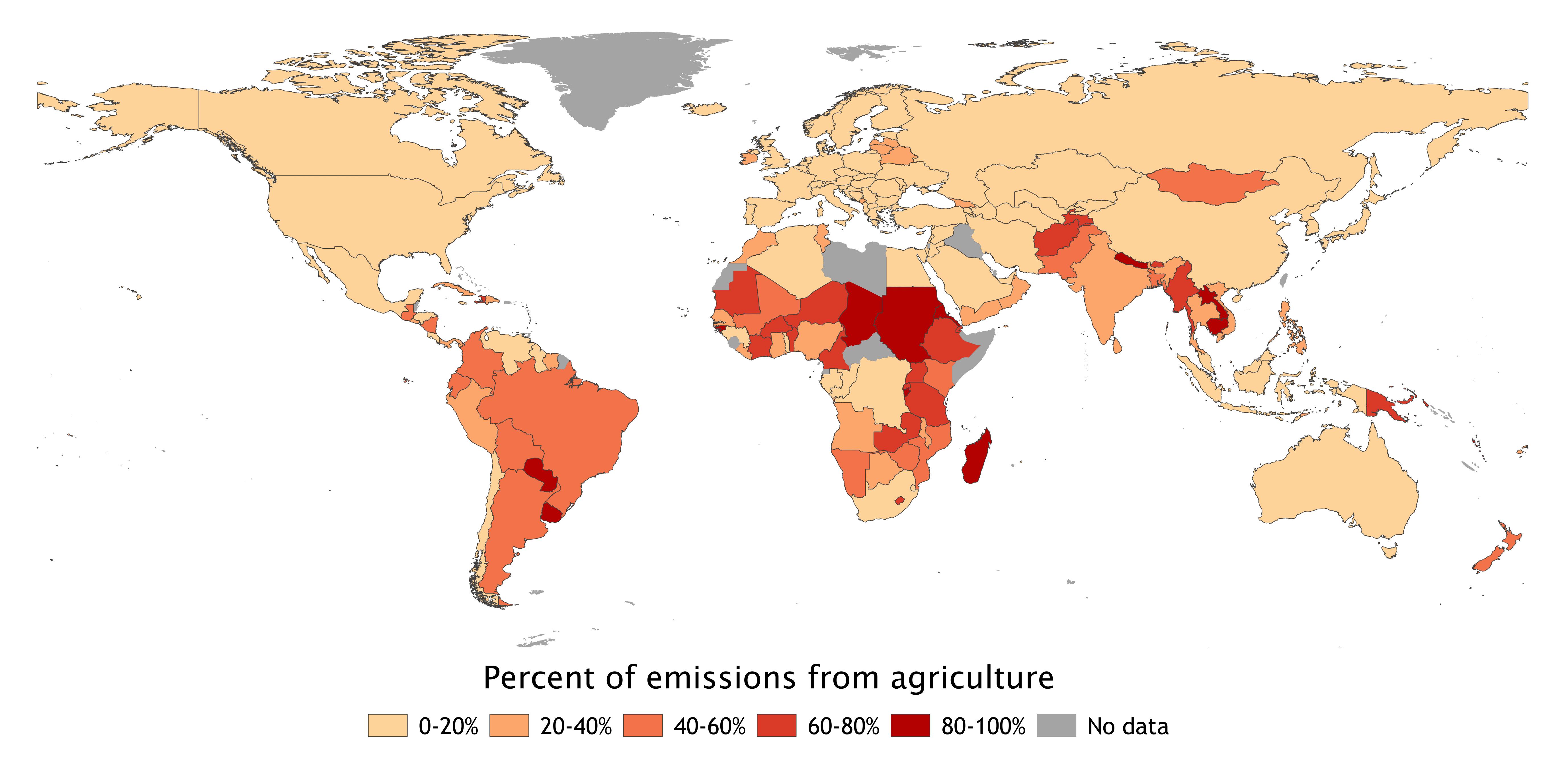
Improved data on agricultural GHG emissions and mitigation potentials provides opportunities to decision makers at all levels. First and foremost, it allows governments and development organizations to identify high production, low-emission development trajectories for the agriculture sector. With the suite of farm- and landscape-level management options for GHG mitigation and improved productivity available for just about any site-specific situation, there are numerous options to select from. Country- or region-specific data allows more accurate comparison of these options. Second, the prospects of the emerging green economy and potential for climate finance will dictate how emission reductions are both valued and verified. Verification, whether for Nationally Appropriate Mitigation Actions (NAMAs) or product supply chain assessments, will require both reasonable estimates of baseline emissions and accurate quantification of emission reductions. Third, economies of tropical developing countries are largely dominated by agricultural production, and this sector contributes a significant fraction to their national GHG budgets (Figure 1.1). Accurate data strengthens the basis for their negotiating position in global climate discussions.
1.2 Who should use these guidelines
These guidelines are intended to inform anyone conducting field measurements of agricultural greenhouse gas sources and sinks, especially to assess mitigation options in smallholder systems in tropical developing countries. The methods provide a standard for consistent, robust data that can be collected at reasonable cost with equipment often available in developing countries. They are also intended to provide end users of GHG data with a standard to evaluate methods used in previous efforts and inform future quantification efforts. The comparative analyses found in these chapters are accompanied by the recommended step-by-step instructions for the methods on the SAMPLES website.
The SAMPLES website (www.samples.ccafs.cgiar.org) provides more detailed manuals and resources for further instruction.
Potential users of the guidelines include:
- – National agricultural research centers (NARS). NARS researchers can use these guidelines to establish protocols for greenhouse gas measurement from agriculture within their institution and ensure comparability with other research partners. They may also be used to review the robustness of existing measurement methods or for finding ways to reduce costs.
- – Compilers of national GHG inventories. These guidelines are intended to provide methods for data collection to support the development of Tier 2 emission factors and the calibration of process-based models for Tier 3 approaches.
- – Developers of national and subnational mitigation plans that include agriculture. Strategies to limit or reduce emissions take multiple forms: Intended Nationally Determined Contributions (INDCs), Low Emission Development Strategies (LEDS), and Nationally Appropriate Mitigation Actions (NAMAs). Accurate information is required both in the planning phase, to establish baselines and compare potential interventions, and in the implementation phase, to monitor, report, and verify (MRV) emissions reductions attributable to the strategy or policy. Field measurements are often necessary to generate national emission factors or calibrate models that can then be used in MRV systems. These guidelines should be used to ensure that field measurements methods are cost-effective, comparable across sites, and of sufficient accuracy.
- – Agricultural commodity companies and agricultural development projects. These guidelines complement greenhouse gas accounting methodologies such as the Product Category Rules (PCRs) and carbon credit standards as well as agricultural greenhouse gas calculators such as EX-Ante Carbon Balance Tool (EX-ACT) (Bernoux et al. 2010) and Cool Farm Tool (Hillier et al. 2011). These methodologies and tools often require, or are improved by, user-input data corresponding to the project area, such as soil C stocks or emission factors for fertilizer application. These guidelines and the associated web resources provide methods—not usually covered in product and project standards—for the field measurements to generate this data
- – Students and instructors. Post-graduate students, advisors, and university instructors can use these guidelines as a manual in selecting research methods.
Box 1.1 Make best use of limited resources by carefully selecting practices for testing
In addition to measurements to establish emissions baselines, GHG measurement is often undertaken with the purpose of comparing mitigation practices. Too often, those practices are chosen randomly or opportunistically, without explicit consideration of their feasibility or mitigation potential. The results of GHG measurement research will be more useful if practices for testing are identified in a systematic way with input from relevant decision-makers. This can be thought of as a process of “filtering” options from a laundry list of potentials to a few for further testing.
Identify the scope of practices for consideration
This can be seen as the “boundary” of potential options. Establishing a spatial boundary is a first step; this may be ecological (a watershed) or political (a county). Additionally, it is useful to further narrow the focus to particular agricultural activities or sectors. The criteria for doing so may include:
- – Extent of an activity within the landscape. The targeting approach described by Rufino et al. (Chapter 2) is useful to determine this, as are agricultural census data and land cover maps.
- – Magnitude of emissions from a given agricultural activity. At the national scale, this can be estimated from FAOSTAT (FAOSTAT 2015), or the national communication to the UNFCCC. At farm or landscape scales, greenhouse gas calculators (Colomb et al. 2013) can provide a rough estimate.
- – Stakeholder priorities. Government development plans and priorities may provide opportunities to incorporate mitigation practices that also improve production or livelihoods. Farmer unions and project funders may have priorities as well. It is good practice to consult a variety of stakeholders in identifying priority activities or sectors, including women and disadvantaged groups.
- – Scale of practice changes to be considered. Different mitigation practices imply differing scales of change within an agricultural system. Some may be incremental practice changes (such as improved nitrogen-use efficiency), whereas others may modify the entire system (such as changing crops or animal breeds, or incorporating trees). Some mitigation options are not “practices” per se, but transformational changes such as different livelihoods or a change in land use, such as changing from nomadic pastoralism to settled agriculture (Howden et al. 2011).
Identify potential practices Once the geography and scope of the mitigation effort have been established, develop a list of practices that may be applicable. Ideas may come from interviews and surveys of stakeholder groups as well as published literature. The website accompanying this volume includes resources for this purpose.
Narrow the list of practices for testing Several criteria should be used to narrow the list of practices to a smaller feasible number for field-testing.
- – Likely mitigation potential. While the purpose of field measurements is to provide accurate information on mitigation potential, expert judgment and currently available emission factors and models can allow a rough estimate to guide field measurements toward practices with the largest potential for reducing emissions. Again, some greenhouse gas calculators are useful for this purpose. The CGIAR Research Program on Climate Change, Agriculture and Food Security is currently developing a tool specifically to rank the most effective mitigation practices in a given geographic area (Nayak et al. 2014).
- – Uncertainty of current information. Sometimes, the most relevant mitigation practice may be one that is already well studied in the project area, or for which uncertainty around mitigation potential is generally low. In these cases, it may be better to focus field measurement efforts on practices for which uncertainty is high, or globally available emission factors are not relevant. If uncertainty has not been quantified, it may be valuable to conduct a small initial measurement effort and compare these results with outputs from available models. This can then guide the larger measurement campaign to areas most needed to reduce uncertainty.
- – Benefits for adaptation and livelihoods. Reduction of greenhouse gas emissions is not the primary focus of farmers or, usually, policy makers. Practices should also be prioritized based on their benefits in terms of productivity, income, and resilience to climate change. Here, input from farmers and their organizations is critical. Likewise, there may be barriers to adoption that make a particular practice impractical or require supportive policies, such as high upfront investment or lack of access to markets (Wilkes et al. 2013).
- – Available Resources. Funding, labor and time will necessarily limit the number of practices for which measurements can be conducted.
1.3 How to use these guidelines
The 10 chapters in this volume are grouped into three categories that correspond with the steps necessary to conduct measurement (1) question definition, (2) data acquisition and (3) ‘option’ identification (synthesis) (Fig. 1.2). Some readers, such as those looking to evaluate mitigation options for an agricultural NAMA, may want to go through each step. Readers interested in measurement methods for a particular GHG source can go directly to the associated chapter.

Step 1. Question definition
Question definition defines the scope, boundaries and objectives of a measurement program. Measurement campaigns may be undertaken for a number of GHG quantification objectives such as developing emission factors, GHG inventories, or identifying mitigation options. The objective has considerable leverage on how and what is measured. In this volume, Rufino et al. (Chapter 2) describes methods for characterizing heterogeneous farming systems and landscapes, identifying the critical control points in terms of food security and greenhouse gas emissions in farming systems and landscapes. This characterization of the system generates fundamental information about the distribution and importance of farming activities in the landscape. Though often overlooked, depending on the preferences and priorities of donors or researchers, systems characterization is critical to target measurements to the most relevant areas in a landscape and stratify the landscape to inform sampling design.
Step 2. Data acquisition
Data acquisition is the ‘nuts and bolts’ of quantification. It represents the activities that are conducted to measure and estimate GHG fluxes or changes in carbon stocks. The six chapters that make up this step discuss methods to quantify stocks, stock changes and fluxes of the major GHG sources and sinks including land use and land cover change (Kearney and Smukler Chapter 3), greenhouse gas emissions from soils (Butterbach-Bahl et al. Chapter 4), methane emissions due to enteric fermentation in ruminants (Goopy et al. Chapter 5), carbon in biomass (Kuyah et al. Chapter 6) and soil carbon stocks (Saiz and Albrecht Chapter 7). Methods to measure land productivity under agriculture—an essential input for tradeoff analysis—are treated separately (Sapkota et al. Chapter 8) (Table 1.1).
Each chapter provides a comparative analysis of existing methods for quanti- fication, particularly evaluating methods across three key features—accuracy, scale, and cost (Table 1.2). Authors provide recommendations about how to select the optimal measurement approaches appropriate to the technical and financial constraints often encountered in developing countries, supplemented with discussion of the limitation of various methods. A central theme of the chapters is that GHG quantification is inherently inaccurate. The biogeochemis- try of the processes that researchers are measuring coupled with the logistical practicalities of research mean that every measurement is only an estimate of the true flux. The researcher must therefore understand how different measurement approaches will affect their estimates and tailor measurement campaigns or quantification efforts to characterize the fluxes necessary to meet program objec- tives in a transparent and objective way. The resultant data on GHG fluxes pro- duced from different sources and sinks can then be aggregated for partial or full GHG budgets using the guidelines from Chaps. 9–10.
Step 3. Estimation of emissions and analysis of mitigation options
The final step is to synthesize the results to identify emissions levels and mitigation options. Data acquisition in Step 2 may take place at multiple scales, ranging from point measurements of individual farming activities (such as soil carbon measurements) to pixel analysis at various resolutions of land use and land cover change. It is then necessary to extrapolate these point measurements of individual features back to scales of interest (fields, farms or landscapes). Rosenstock et al. (Chapter 9) describe the three principal ways that this can be accomplished: empirical, process-based models or a combination of both. Van Wijk et al. (Chapter 10) provide guidance on approaches to synthesize all the data in the production of estimates of tradeoffs or synergies in various farm or landscape management activities. Tradeoff analysis, though originating in the 1970s, has been developing rapidly due to increase in computing power and advances in theory and modeling frameworks. However, the authors stress that practical analysis has to include stakeholders to integrate their own perspectives and preferences for the analysis to be practically valuable. By developing estimates of GHG fluxes at relevant scales and analyzing tradeoffs, the approaches detailed in this volume can inform for low-emissions development planning.
Sample Chapter | 1996 IPCC Guidelines | 2006 IPCC Guidelines |
Chapter 3: Determining GHG emissions and removals associated with land use and land cover change | 5 Land use change and forestry | 3B Land |
Chapter 4: Measuring GHG emissions from managed and natural soils | 4C Rice cultivation 4D Agricultural soils | 3C2 Liming 3C3 Urea application 3C4 Direct N2O emissions from managed soils 3C5 Indirect N2O emissions from managed soils 3C7 Rice cultivations |
Chapter 5: Measuring methane emissions form ruminants | 4A Enteric fermentation | 3A1 Enteric fermentation |
Chapter 6: Quantifying tree biomass carbon stocks and fluxes in agricultural landscapes | 5A Changes in forest and other woody biomass stocks 5B Forest and grassland conversion 5C Abandonment of managed lands 5-FL Forest land 5-CL Cropland 5-GL Grassland | 3B1 Forest land 3B2 Cropland 3B3 Grassland |
Chapter 7: Methods for quantification of soil carbon stocks and changes | 5B Forest and grassland conversion 5C Abandonment of managed lands 5D CO2 emissions and removals from soil 5-FL Forest land 5-CL Cropland 5-GL Grassland | 3B2 Cropland 3B3 Grassland |
Chapter 8: Yield estimation of food and non-food crops in smallholder production systems | 4F Field burning of agricultural residues (for calculating residue quantities) | 3C1b Biomass burning on croplands |
Table 1.2 Examples of measurements options and their accuracy, cost and scale implications based on analysis in this volume.
The images or other third party material in this chapter are included in the work’s Creative Commons license, unless indicated otherwise in the credit line; if such material is not included in the work’s Creative Commons license and the respective action is not permitted by statutory regu- lation, users will need to obtain permission from the license holder to duplicate, adapt or reproduce the material.
Acknowledgements
The framework of these guidelines was developed during a global workshop of experts on greenhouse gas quantification in Garmisch-Partenkirchen, Germany in October 2012.
Participants:
-Alain Albrecht, Institut de Recherche pour le Développement (IRD), France
-Andre Butler, IFMR LEAD, India
-Aracely Castro Zuñiga, Consultant – Soils Research & Management at FAO, Italy
-Ngonidzashe Chirinda, International Center for Tropical Agriculture (CIAT), Colombia
-Alex DePinto, International Food Policy Research Institute (IFPRI), USA
-Jonathan Hickman, Columbia University, USA
-ML Jat, International Maize and Wheat Improvement Center (CIMMYT), India
-Brian McConkey, Agriculture and Agrifood Canada
-Ivan Ortiz Monasterio, International Maize and Wheat Improvement Center (CIMMYT), Mexico
-Barbara Nave, BASF, Germany
-An Notenbaert, International Livestock Research Institute (ILRI), Kenya
-Susan Owen, Center for Ecology and Hydrology, UK
-J Prasad, Central Research Institute for Dryland Agriculture (CRIDA), India
-Meryl Richards, University of Vermont and CGIAR Research Program on Climate Change, Agriculture and Food Security (CCAFS), USA
-Philippe Rochette, Agriculture and Agrifood Canada
-Todd Rosenstock, World Agroforestry Centre (ICRAF), Kenya
-Mariana Rufino, Center for International Forestry Research (CIFOR), Kenya
-B Ole Sander, International Rice Research Institute (IRRI), Philippines
-Sean Smukler, University of British Columbia, Canada
-Piet van Asten, International Institute of Tropical Agriculture (IITA), Uganda
-Mark van Wijk, International Livestock Research Institute (ILRI), Costa Rica
-Jonathan Vayssieres, CIRAD, Senegal
-Lini Wollenberg, University of Vermont and CGIAR Research Program on Climate Change, Agriculture and Food Security (CCAFS), USA
-Xunhua Zheng, Institute of Atmospheric Physics- Chinese Academy of Sciences (IAP-CAS), China
We would also like to acknowledge the following individuals who provided feedback on all or part of the guidelines during the review process:
-Juergen Augustin, Leibniz Centre for Agricultural Landscape Research
-Rolando Barahona Rosales, National University of Colombia (Medellín), Colombia
-Ed Charmley, Commonwealth Scientific and Industrial Research Organisation, Australia
-Nicholas Coops, University of British Columbia, Canada
-Nestor Ignacio Gasparri, National University of Tucumán, Argentina
-Jeroen Groot, Wageningen University and Research Centre, Netherlands
-Ralf Kiese, Karlsruhe Institute for Technology, Germany
-Carlos Ortiz Oñate, Technical University of Madrid, Spain
-Philippe Rochette, Agriculture and Agri-Food Canada
-Sileshi Weldesmayat, World Agroforestry Centre, Kenya
-Jonathan Wynn, University of South Florida, USA
Author affiliations
¹ Todd S. Rosenstock, World Agroforestry Centre, PO Box 30677-00100, UN Avenue-Gigiri, Nairobi, Kenya, t.rosenstock@cgiar.org, +254 20 722 4838
2 International Rice Research Institute (IRRI)
3 International Livestock Research Institute (ILRI)
4 Karlsruhe Institute of Technology (KIT)
5 Lancaster Environment Centre, Lancaster University, formerly Center for International Forestry Research (CIFOR), Nairobi, Kenya
6 Earth Institute, Columbia University
7 International Maize and Wheat Improvement Centre (CIMMYT)
8 Gund Institute for Ecological Economics, University of Vermont, Burlington
9 CGIAR Research Program on Climate Change, Agriculture, and Food Security (CCAFS)
References
IPCC (1996) Revised 1996 IPCC Guidelines for National Greenhouse Gas Inventories. OECD, Paris
Nayak D, Hillier J, Feliciano D, Vetter S. CCAFS-MOT: a screening tool. Presented at a learning session during the 20th session of the Conference of the Parties to the United Nations Framework Convention on Climate Change, Lima, Peru, 3 December 2014. http://ccafs.cgiar.org/mitigation-options-tool-agriculture. Accessed 10 April 2015